
Enterprise Data Strategy & AI: Lessons and Insights for Energy Retailers
Gorilla was founded for a simple reason: energy retailers were not utilising their data to the fullest and needed help. Since then, there has been a lot of progress from retailers seeking to expand their data portfolios, but external issues have magnified the challenges facing utilities. The drive towards net zero has accelerated as nations seek to grapple with the looming threat of climate change. Progress has been disrupted by the energy price shocks we have seen over the past few years, with volatility still high.
This article follows our presence at one of the biggest energy events in Western Europe, E-World in Essen, Germany. The German market has undergone particular stress, with government initiatives to cut back on nuclear coinciding with the cutoff from Russian natural gas following the invasion of Ukraine. At the same time, energy retailers in the country are playing catch up with more advanced markets in the rest of Europe - even now, retailers are struggling to roll out smart meters on a wide enough scale.
Putting AI implementation on top of all the other challenges that energy CIOs are facing is a lot to ask. How will the new wave of machine learning AIs help CIOs to solve their data issues and deal with the wider problems coming in the energy industry? Is there room for AI to play a major role in your data strategy or wider technology strategy? What do the experts at Gorilla recommend? Let’s find out.
Data is your new key function
The title of this article talks of “data strategy”, but the growing importance of data management means it’s probably more accurate to talk of data as a new business function, equivalent to IT, finance, or marketing. It is quite possible that future business leaders will see data as a cost centre, led by a Chief Data Officer or VP, and considered a key strategic asset. Most businesses are not quite there yet, nor will every industry have the same approach to data, but there is no denying the growing importance of data.
The biggest problem facing this potential function? Lack of expertise. People very rarely specialise in data, and those that do often purely from a technical perspective. Management of data has always simply been a subtask for CIOs and other IT leaders, not an area demanding years of specialist experience. Trying to manage a rapidly changing area without having that base of knowledge will strain many.
The growth in data for energy retailers has not followed other industries, where the internet was the biggest driving force. Instead, liberalisation and advances in technology have gone hand in hand. When energy production, distribution, transmission, and retail were all under one entity, the tasks facing utilities were smaller, despite the additional responsibilities. As each part has been split up, complexity has increased, and the number of potential data points has grown exponentially. What was manageable with legacy technologies even 10 years ago is now far beyond the capabilities of undeveloped IT portfolios. Companies are going to be forced to make changes whether they like it or not.
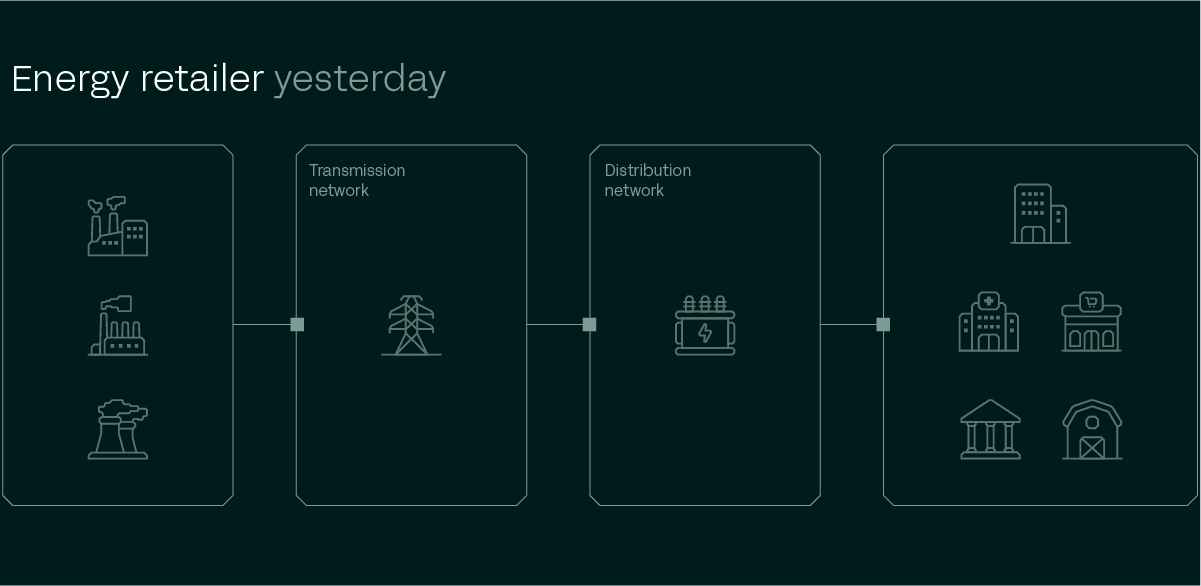
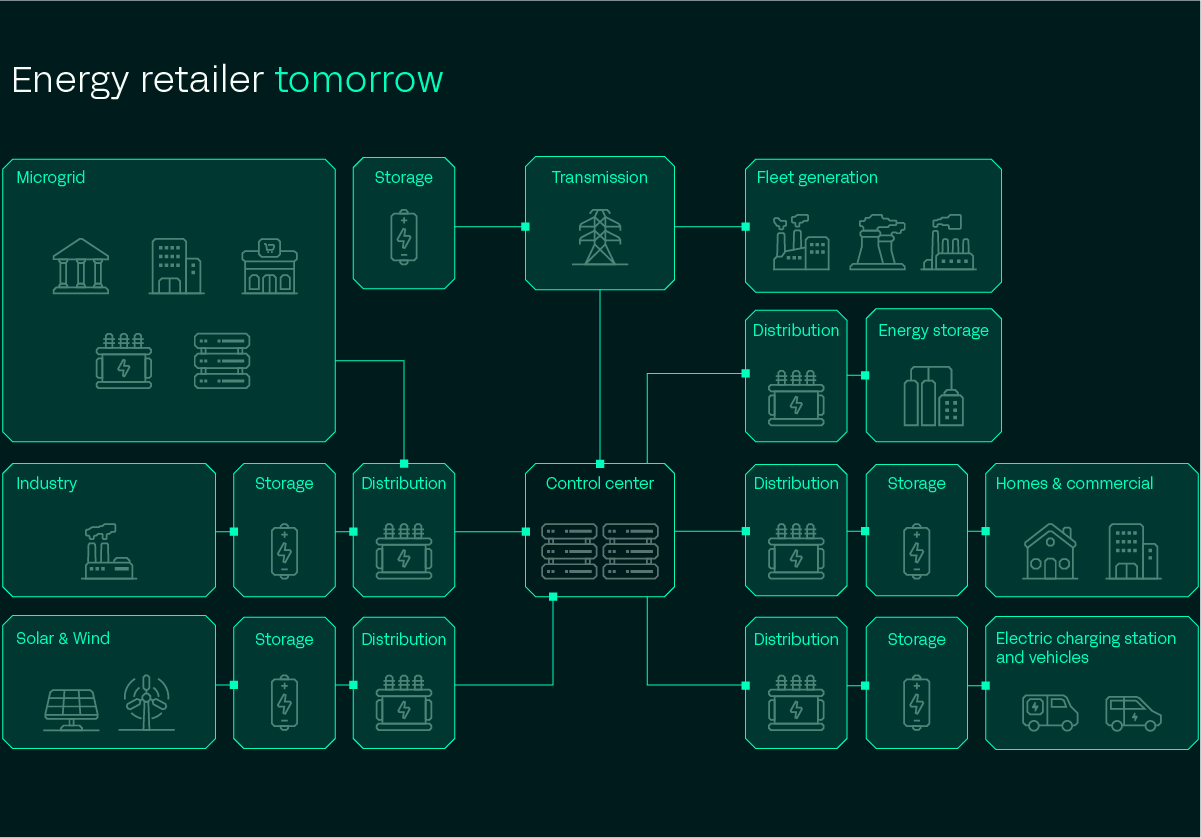
Orchestration of all of so many activities would be resource intensive for any industry, but energy faces the unique challenge of needing to do it in real time, placing huge strain on your data processing capabilities. Automation of everyday activities will be essential to deliver results, requiring a large change to approaches that have traditionally been highly manual and reliant on highly skilled human input.
This puts energy CIOs in a difficult position. Even in energy markets that are less mature, leaders will be well aware of how important data is going to be. At the same time, few in energy will know the best approaches for managing data or planning for the long term, and there is not a large supply of external hires. Into this mix comes the new interest in AI. Could AI be a lifeline for companies that don’t know how to handle their data? Or will it prove to be one more complication?
The AI Opportunity
Every CIO will have their own projects they are keen to advance, but the upheaval described above provides the opportunity for other stakeholders to advance AI as the new solution to all the company’s problems. Even those CIOs skeptical about the short or medium term benefits of AI will need to have a strong case for why any major changes to their technology portfolio should not plan to incorporate AI.
This begs the question as to the possible role that AI can play for energy retailers. Much of the recent hype has arisen from machine learning algorithms, specifically large language models (LLMs) such as OpenAI’s GPT. While these AIs promise to improve productivity in many areas, it is not clear what chatGPT, or Bard, or Midjourney, might do for a utility.
If anything, the lack of clarity over what AI means is one barrier. There are many applications and functions within applications that can be called AI, but they will have vastly different capabilities. Autocorrect in a mobile phone and ChatGPT can both be called artificial intelligence and both generate text, but it is safe to say that no businesses are preparing for the dramatic impact of the former. It might be better to refer to Machine Learning, or ML, as the area that stakeholders will be interested in.
Language Models present an immediate opportunity for user experience improvements, such as through chatbots - though caution is also needed here as this story demonstrates. There is also the potential for general productivity gains, for example with AI-assisted writing for sales or embedded AI in business applications.
Energy retailers may make use of language models for customer support or sales, but they won’t have any success deploying them for data processing. That being said, machine learning has been applied to data and statistics to some success. In fact, these models have been around a lot longer than the recent boom in LLMs; their results simply don’t have the wow factor that a ChatGPT does. This is likely where utilities will see the most potential value from investments in ‘AI’. Predictive analytics, ML, and other AI methods are used to identify trends, clusters, and other patterns in data. This can lead to augmented or automated decision making. Other insight areas that benefit from AI include models for optimizing, forecasting, personalization, or fraud detection.
Could retailers train their own models using ML? The data available to energy companies does seem to present an opportunity to apply machine learning techniques. The likes of AWS and Azure can offer ML services to get around the lack of compute. However, there are still some large barriers. The average utility is not going to be filled with Tensorflow experts or anyone capable of building the algorithms. The amount of data is going to be a limiter, and will need a lot of work on cleaning and analytics before it is ready to be used. And that is assuming that government regulation does not get involved and limit the use of customer data for training purposes.

Each approach to unlocking the potential of AI will have its advantages and disadvantages, as the image above shows. The majority of retailers are in the “Buy” area, lacking at this time the talent or use case to build, but internal machine learning solutions could be one to look at in the long-term. If there are any lessons to be applied to your data strategy now, it is that preparation will be essential. Getting it right today will open up new avenues tomorrow, even if this doesn’t satisfy stakeholders looking for immediate change.
Data Strategy
Even if a game-changing AI tool was ready for energy retailers in the near future, should that change your approach? When it comes to IT or data strategy, technology should not be the driving force. Technology should fit around your goals and objectives rather than trying to fit the company around technology.
How should you approach your enterprise data strategy, if AI is not the answer? As mentioned above, one approach is to start building towards AI, creating the capabilities necessary for machine learning, but this approach won’t be for everyone. Some will be nervous of regulation, or simply won’t trust handing over key parts of their business to AI. Others will turn to third-party suppliers rather than try to do everything in-house.
AI is just one small part in a much larger data strategy which should be enabled when it can add benefits. However, if the larger data ecosystem is not set up it will be complex to achieve true sustainable benefits for the long term as ML is hard to operationalise at scale. These issues are compounded by the problem of trust: business leaders simply won’t be able to trust the output of AI unless it has a consistent track record, but it can be hard to do that without trust.
Looking away from AI, what can we recommend? There isn’t going to be a one-size-fits-all approach as each company and market is just too different, but there are enough commonalities that we can recommend a few steps everyone should take.
Below is an example of a simplified data ecosystem for an energy retailer, featuring Gorilla’s platform. Not every company will need every aspect for their data strategy, but it’s a good starting point.
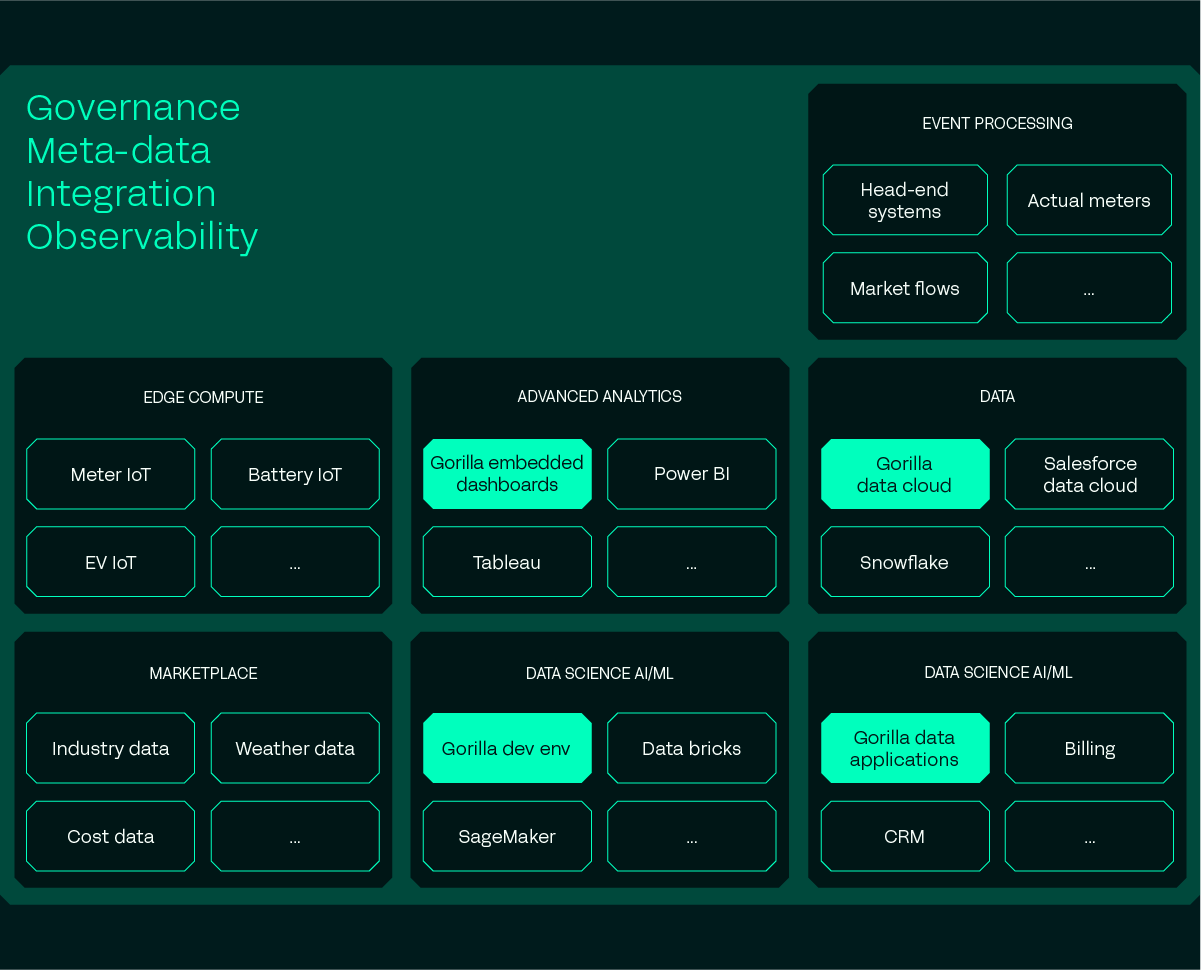
- Invest in data first
The biggest lesson to take from the above illustration is to examine how your current data capabilities measure up, and consider what investment might be needed to reach this level. By making data a priority for investment, you can use the data ecosystems to resolve distributed data challenges by improving their ability to reach beyond their primary business area and to integrate with data sources outside of their immediate domain. Find ways to make data available and usable beyond their source domain. Focus on different integration patterns that are based on shared data governance and meta-data to achieve this.
- Be ready for multiple scenarios
The spread of data monitoring tools and sensors has implications beyond just metering customers or load balancing. Being able to predict weather patterns or distribution disruptions is now feasible for utility companies, permitting a move towards adaptive supply that reacts to events before they occur. However, even this additional data will not produce 100% accurate forecasts - to be truly proactive retailers will need to generate scenarios for every potential situation. With older, manual ways of working this would never be possible, but modern tooling can handle it - but this is assuming you do have a workable ecosystem as illustrated. IoT and external marketplace connectors provide the data. Your data cloud will handle integration and access, which provides the flexible data access for data analytics tools to produce valuable results.
- Put your insights into action
Leverage either your current applications or top-tier, specialized SAAS platforms to apply your insights automatically and on a large scale throughout your operations. Make sure your integration, governance, and meta-data enable you to utilize all pertinent data and automate the insights and models developed by your analysts. It's crucial to note that these applications will generate additional meta-data, which can further enhance your long-term insights and understanding.
- Invest in real-time data available to manage load orchestration
Intermittent sources of energy and constraints on energy sources have both contributed to the need for greater load resilience to ensure consistent supply to customers. Real-time data platforms will be essential to deliver load orchestration that can ensure load security efficiently without the need for manual effort. For residential suppliers, companies will need the ability to scale across millions of devices in real time to deliver promised capabilities. Commercial and Industrial supply will not be at quite that scale but you will still need to invest to get real-time results. Will you utilize a data streaming platform like Apache Kafka or Pulsar? What about storage? Do you need a data lake or data warehouse? Traditional ETL pipelines are not going to be enough for genuine real-time analysis and decision making.
Final Word
This article has been somewhat dismissive of artificial intelligence, but if we have learned anything over the last few years it is that the pace of development can shock even insiders. We have used terms like short and medium term, but in reality we can’t be certain of AI capabilities even on a 6 month timeline, let alone over the years that might constitute medium term. Perhaps the most important strategic takeaway is to be ready for dramatic change and to be capable of shifting direction rapidly.
Even if developments are not that dramatic, it is already past time to start preparing for the impact that AI can have. You need to get your data ecosystem up and running before any value can be delivered through it. You will need good clean data and governance over that data to get trustworthy results you can use, whether they are processed by AI or other tools.
At the same time, there are more mundane challenges that need to be addressed. IoT and other sensors are delivering a much greater volume of external data. Intermittent energy generation and changes to the demand profile will place much greater pressure on load resilience. The advantage that CIOs have is that in many cases the answers to these questions, as well as to AI, require the same solutions. Modern data ingestion & edge compute, real-time streaming & processing, data cloud, and other data technologies will all contribute to the creation of a complete data ecosystem.
Gorilla is one such data platform that can form a building block. Cloud-based data transformation will form a key component in between your storage and ingestion solutions. Gorilla provides an automated, auto-scaling platform delivering analytics and applications for areas such as pricing, forecasting, and portfolio analysis. Request a demo today to find out how Gorilla can empower your data strategy.